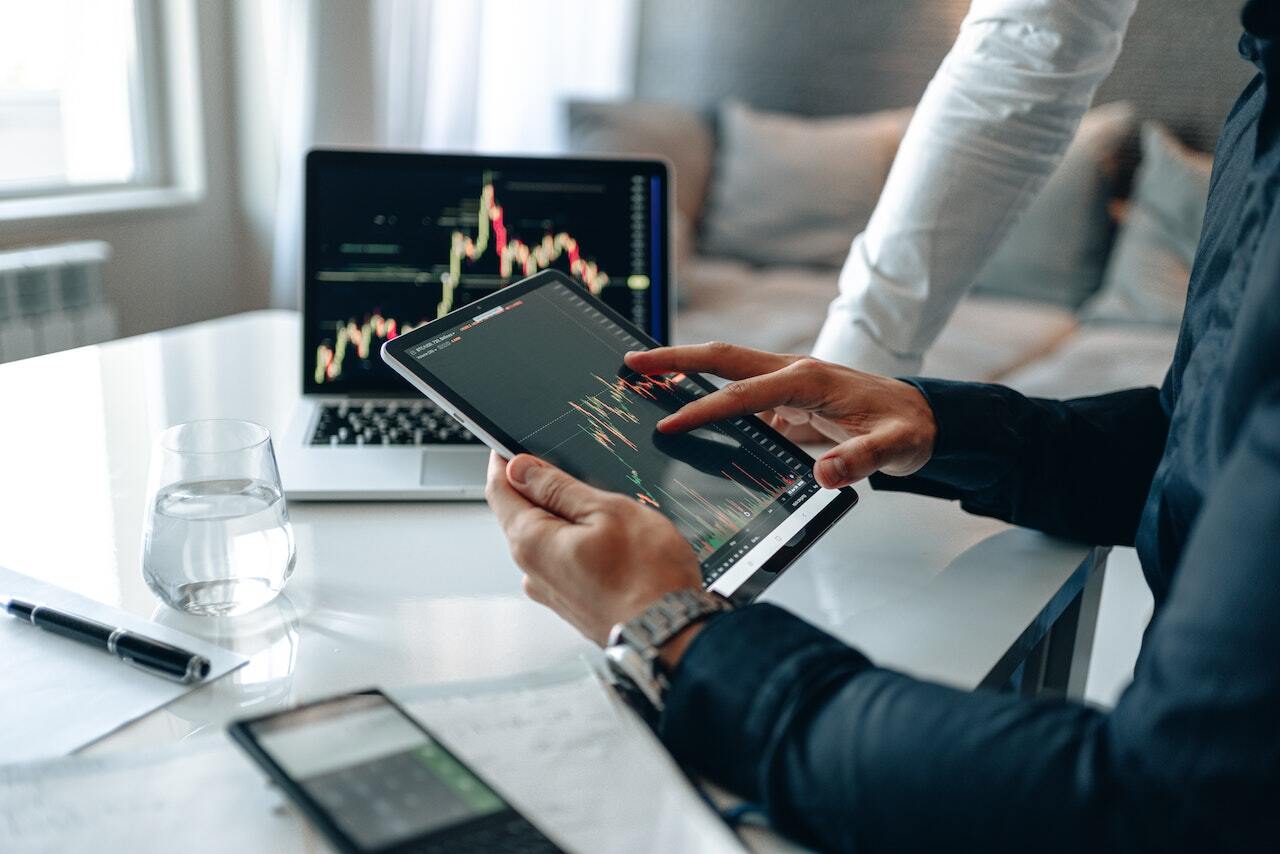
The idea of a Data Mesh has emerged as a strategy, for managing complex and distributed data systems. As organizations grapple with volumes of data coming from sources and being used by different teams ensuring data observability has become a crucial challenge.
Data observability involves tracking, monitoring and understanding the flow of data through pipelines. The Data Mesh architecture seems to align with this need offering improved visibility and comprehension of data. This article explores how the principles of data observability and the Data Mesh model intertwine to provide enhanced insights into data.
What is Data Observability?
Data observability draws inspiration from software observability and applies it to the domain of data. Similar to how software observability aims to provide insights into the state of software systems data observability focuses on gaining insights into the state and behavior of data pipelines.
This entails tracking the movement of data monitoring its quality and understanding its transformations. Traditional monolithic data architectures often struggle to achieve observability due to their nature making it challenging to keep track of data as it moves across different stages.
Data Mesh – An Introduction:
The Data Mesh approach tackles the challenges presented by centralized data architectures, by prioritizing domain-oriented decentralization. Data Mesh operates by considering data domains as entities with each domain taking ownership of a business domain.
This approach encourages a sense of ownership and responsibility as individual teams specializing in domains gain an understanding of the unique aspects of their data. Of relying on a centralized data pipeline, the Data Mesh framework suggests the implementation of domain specific data pipelines that can be developed deployed and managed independently by each respective team.
The Convergence: Where Data Observability Meets Data Mesh
At glance data observability and the Data Mesh concept may appear to be ideas. However, they complement each other in ways to provide an integrated solution, for managing data ecosystems. Let's delve into how they work:
1. Granular Monitoring and Tracking:
Within a Data Mesh framework each domain operates its data pipeline. This inherently enables tracking of the entire lifecycle of data. With domain pipelines in place, it becomes easier to monitor how data flows from its source to its destination. This level of granularity enhances observability by offering insights into the performance and overall health of domains datasets.
2. Insights into Data Quality:
Data observability encompasses aspects related to data quality well. The Data Mesh approach promotes the idea of treating each domains produced data as a product with defined quality standards. By adopting this mindset shift maintaining high quality data starts at its source within each domain itself. Subsequently tools, for data observability can focus on evaluating whether these produced datasets meet the predefined quality thresholds.
3. Understanding Context:
Having context is crucial, for understanding data. Traditional data architectures often lack context as data moves from one stage to another. In a Data Mesh domain teams understand their data, its meaning and how it relates to their business domain. This deep knowledge greatly contributes to an understanding of the data.
4. Decentralized Troubleshooting:
In architectures where data pipelines span across domains identifying the root cause of issues can be complex. The decentralized nature of the Data Mesh simplifies debugging. If a problem occurs it's easier to pinpoint the domain streamlining troubleshooting efforts.
5. Flexibility and Iteration:
Data Mesh promotes agility by allowing domains to iterate on their data pipelines independently. This iterative approach aligns with the concept of observability, where continuous refinement and adaptabilities crucial for maintaining a view of how data flows.
Final Thoughts:
In todays era of data driven decision making having observability, over your data is not a luxury but a necessity. The Data Mesh architecture presents itself as a solution that aligns with the principles of ensuring observability.
By decentralizing data domains and fostering ownership the Data Mesh enables monitoring, quality control, contextual understanding, decentralized troubleshooting and iterative development.
The convergence eventually leads to visibility and comprehension of data landscapes.
As companies navigate through the network of data embracing the beneficial relationship, between Data Mesh and data observability might be the solution, to uncovering valuable insights hidden within the data.