.jpg)
Gen AI is the buzzword word right now and its widespread popularity can be attributed to its crucial benefits and characteristic features that heavily impact routine tasks, communications, and production. Generative AI is an emerging field that is slowly driving many businesses in different industry sectors today.
In this article, we delve deep into the topic by exploring right from the Gen AI definition, benefits, features to its downside. So, continue to read on...
What is Generative AI?
Generative AI is a facet of artificial intelligence technology that can craft diverse forms of content, spanning from text, imagery, and audio to synthetic data.
The sudden fame encompassing generative AI emanates from the user-friendly interfaces introduced recently, enabling the swift creation of top-notch text, graphics, and videos within seconds. These interfaces leverage the potency of generative AI models, wherein the AI system comprehends the patterns and arrangement within the provided training data, subsequently producing fresh data imbued with akin attributes and qualities.
Why Generative AI Matters?
Ever since Generative AI started off in full-swing, business leaders have been pushed to deal with three new sets of challenges:
- Investors anticipate more improved margins and new sources for robust growth.
- The use of generative AI (Gen AI) in daily life is increasing, and thereby, customers expect businesses to use it proactively.
- Effective cost-cutting through replacing humans with Gen AI for accomplishing routine jobs.
As a result, the responsibility falls on the business leaders and corporate heads to figure out:
- How Generative AI can fit into current and potential business and operating models?
- How to effectively experiment with Gen AI use cases?
- How to get ready for the longer-term disruptions and possibilities brought on by Gen AI trends?
Generative AI - Examining its origins
Gen AI technology is not all new in the science world, it was first introduced in the 1960s in chatbots. However, it was not until 2014 that generative adversarial networks, or GANs a type of machine learning algorithm emerged, allowing Generative AI to convincingly create authentic images, videos, and audio of real individuals. On one hand, this newfound capability has unlocked opportunities like improved movie dubbing and enriched educational content.
Simultaneously, it has also raised concerns about deepfakes, which are digitally manipulated images or videos, and cybersecurity threats, including malicious requests realistically mimicking an employee's superior. The realm of machine learning frequently employs statistical models, including generative models, for data prediction and modeling. Progressing from the late 2000s, the rise of deep learning propelled advancements in tasks like image classification, speech recognition, and natural language processing. In this phase, neural networks primarily functioned as discriminative models due to the complexity of generative modeling.
In 2014, breakthroughs like the variational autoencoder and generative adversarial network yielded the first functional deep neural networks capable of learning generative models for intricate data, such as images. These deep generative models became the pioneers in producing not only class labels for images but entire image outputs. The Transformer network's emergence in 2017 led to progress in generative models, culminating in the debut of the first generative pre-trained transformer (GPT) in 2018. Subsequently, GPT-2 in 2019 showcased its unsupervised generalization across diverse tasks as a foundational model.
By 2021, the introduction of DALL-E, a transformer-based pixel generative model, along with Mi journey and Stable Diffusion, marked the rise of high-quality artificial intelligence art generated from natural language prompts. In March 2023, the release of GPT-4 took place, with Microsoft Research arguing that it could be seen as an early (albeit incomplete) version of an artificial general intelligence (AGI) system.
Generative AI Journey Through The Years...
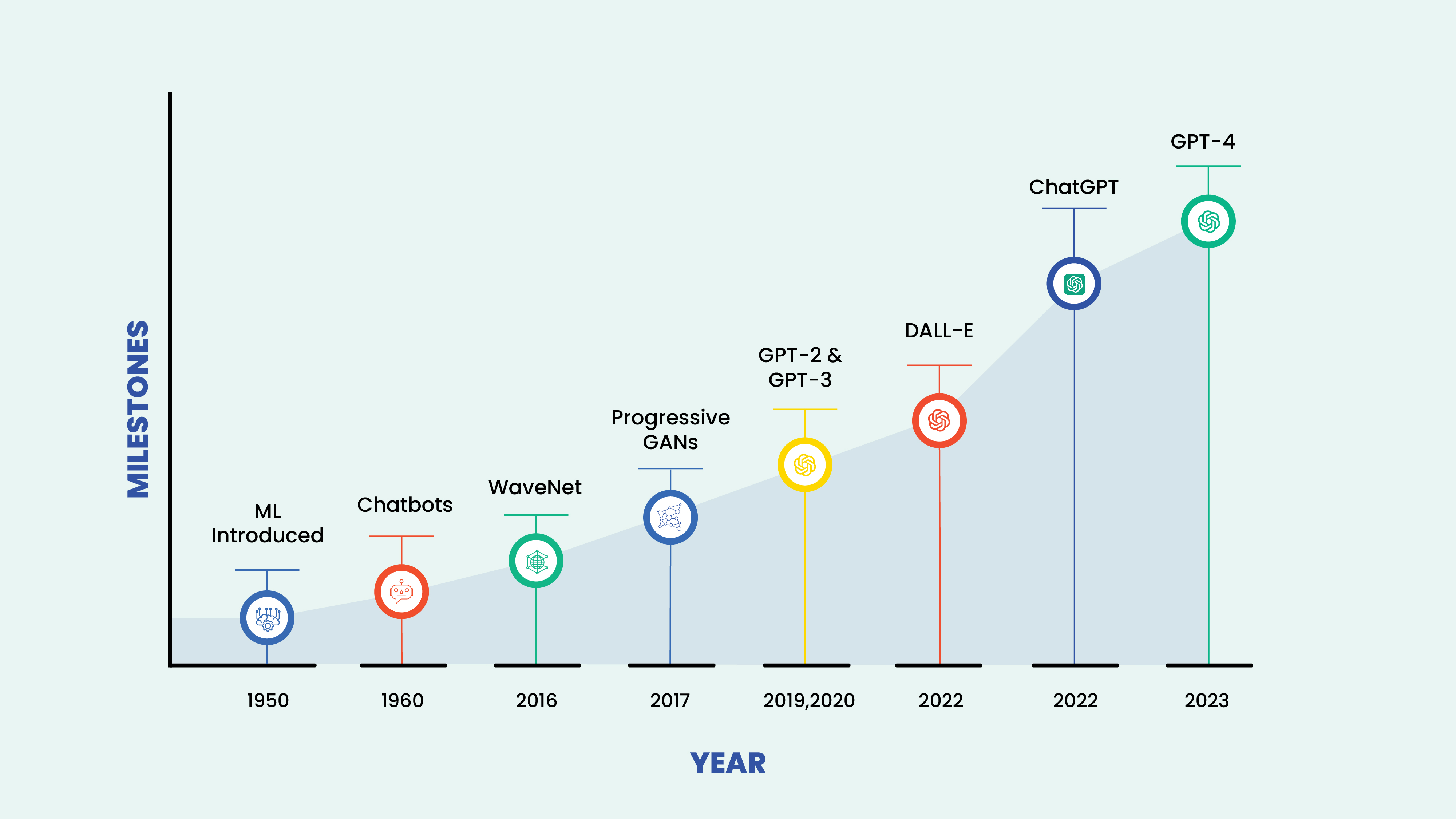
What are foundation models in Generative AI?
Foundation models in Generative AI refer to the large-scale trained neural network models that form the foundation for a wide range of tasks related to understanding and generating natural language. These models are usually trained on datasets that contain internet text allowing them to learn and understand language patterns and knowledge. Foundation models play a pivotal role in applications of natural language processing (NLP).
The primary characteristics of foundation models include:
1. Pre-training: Foundation models go through a training phase where they learn from a large amount of text data. During this phase, the model comprehends to predict words in a sentence based on the context provided by preceding words. This pre-training helps the model to develop an understanding of language and common-sense knowledge.
2. Transfer learning: After pre-training, these models can be fine-tuned for tasks using datasets tailored for those tasks. Fine-tuning allows the model to adapt its acquired knowledge to perform tasks such, as text classification, language translation, text generation, sentiment analysis, and similar applications.
3. Scale: Foundation models are well known for their size often consisting of hundreds of millions or even billions of parameters. The extensive size of these models enables them to capture nuances in language and excel across tasks.
4. Generalization: The advantage of training models, on different sources of data is that they can easily adapt to different language tasks without needing a large amount of task-specific training data.
5. Accessibility: Foundation models that have been trained are frequently made available to the public by organizations such, as OpenAI, Google, and Facebook. This accessibility has enabled researchers and developers in the field of NLP to create language-based applications without having to start from square one.
Generative AI models with examples
AI models that generate content utilize algorithms to process and represent data. For example, when it comes to text generation different methods, in natural language processing are employed to convert characters like letters, punctuation, and words into sentences, parts of speech, entities, and actions. These are then transformed into vectors using encoding techniques. Similarly, images transform to capture elements represented as vectors. It's worth noting that these techniques might have biases, racism, deception, or exaggeration present in the training data.
Once developers decide on the approach for representation, they employ networks to generate fresh content in response to queries or prompts. Techniques like GANs (Generative Adversarial Networks) and variational autoencoders (VAEs) which consist of a decoder and an encoder component can produce faces or synthetic data for AI training purposes. They can even imitate individuals if desired.
Recent advancements in transformers have also brought about networks capable of encoding language (such as Google's Bidirectional Encoder Representations from Transformers. BERT) images (like OpenAIs GPT) and proteins (such, as Google AlphaFold). These transformers are not only able to encode information but also generate content.
Dall-E, ChatGPT and Bard – what are they?
Of late, Dall-E, ChatGPT, and Bard have earned a significant name and popularity as one of the best Generative AI interfaces. Let's look at all three respectively:
Dall-E:
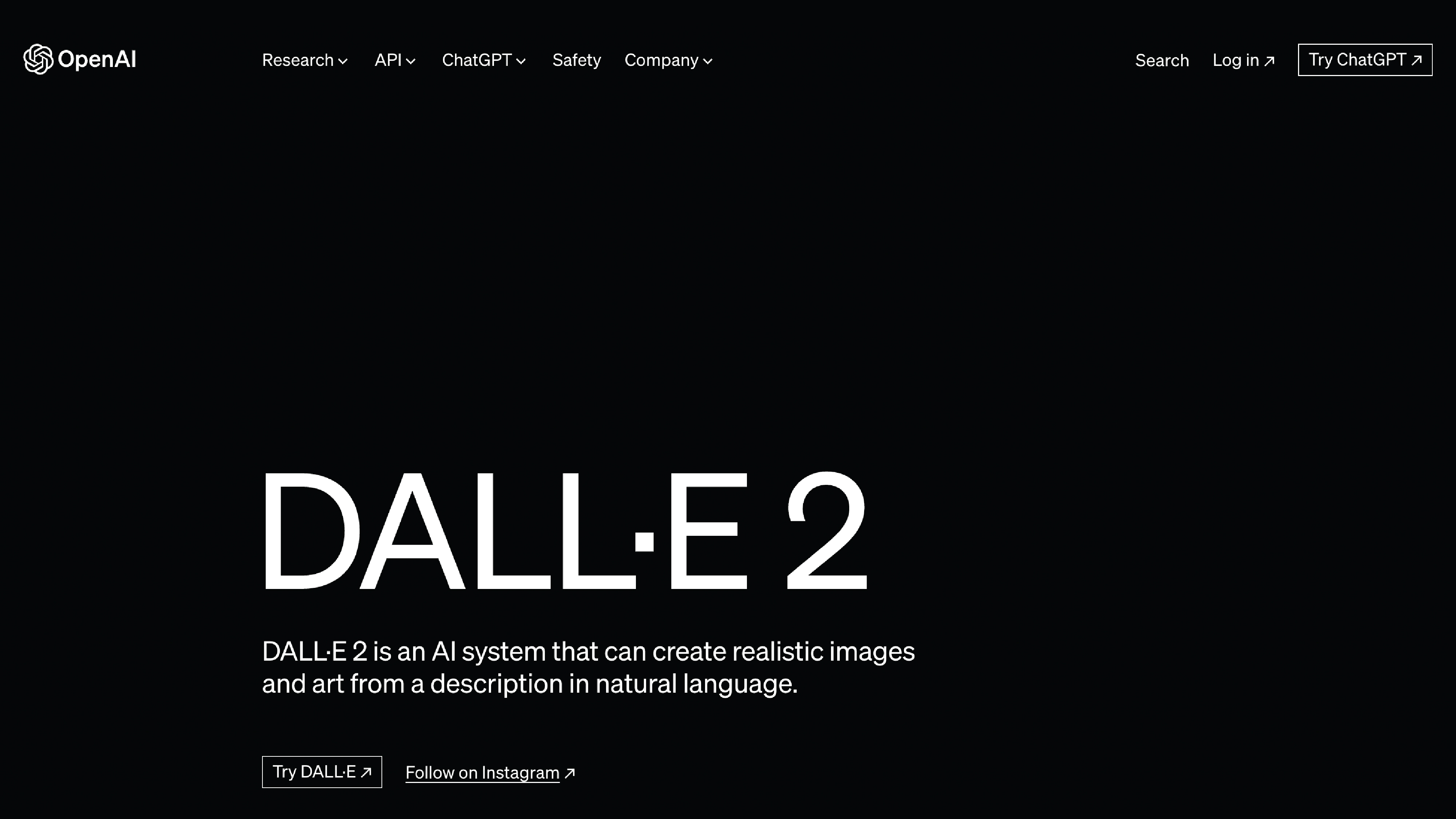
Dall-E, an instance of multimodal AI, was developed by training on an extensive dataset comprising images and their corresponding textual descriptions. It excels at establishing links across diverse media forms, encompassing vision, text, and audio. Specifically, it links word meanings to visual components, employing OpenAI's GPT architecture introduced in 2021. An enhanced iteration, Dall-E 2, was introduced in 2022, expanding its capabilities to empower users to produce diverse imagery styles guided by their prompts.
ChatGPT:
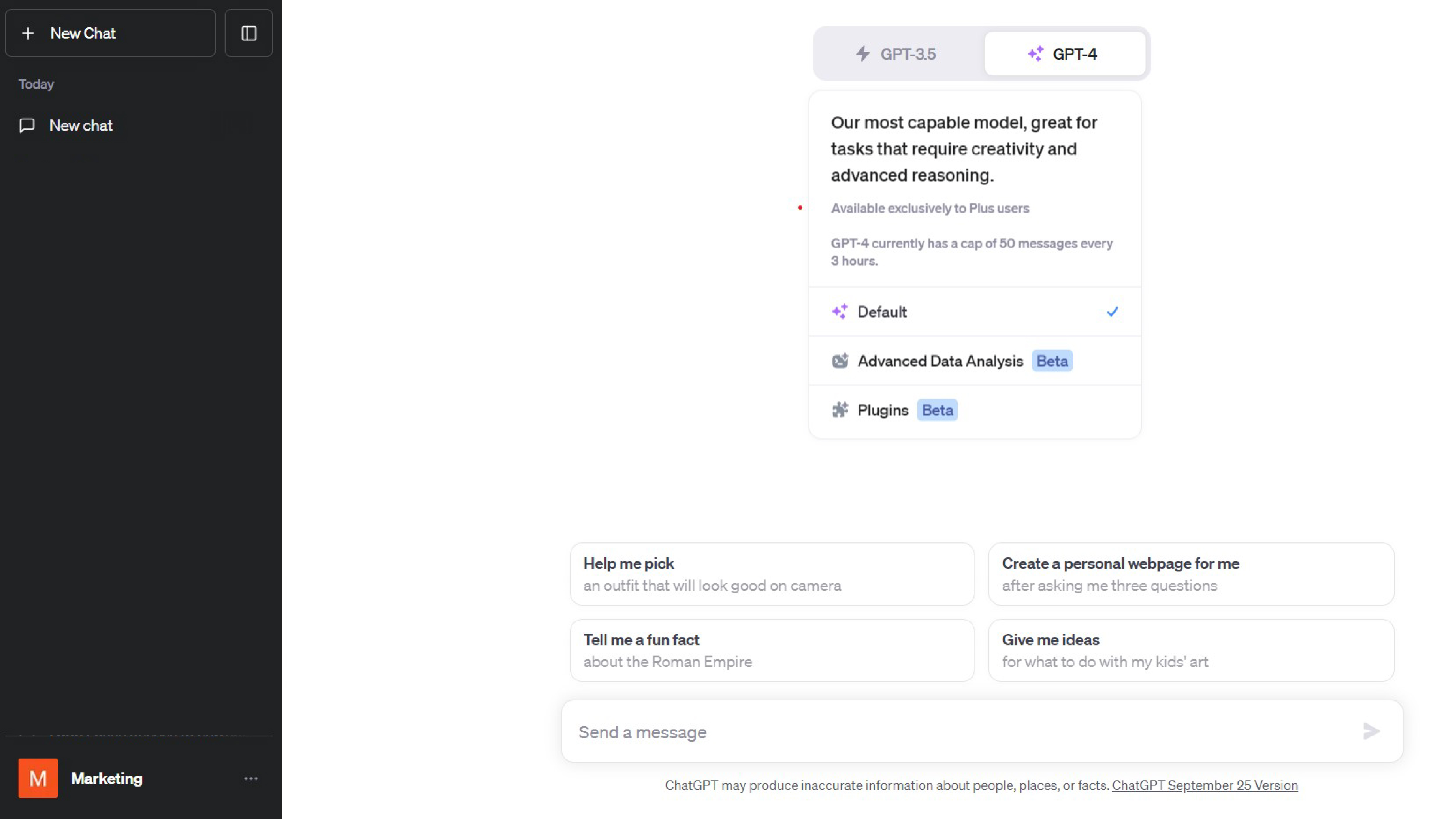
In November 2022 the world was captivated by a chatbot powered by AI specifically based on OpenAIs GPT 3.5 model. OpenAI introduced a method to interact and improve text responses through a chat interface that allows for feedback. Unlike versions of GPT, which were only accessible, via an API GPT 4 made its appearance on March 14, 2023. ChatGPT seamlessly incorporates conversations with users creating an interaction experience. Due to the success of this GPT interface, Microsoft invested significantly in OpenAI and integrated a variant of GPT into its Bing search engine.
Bard:
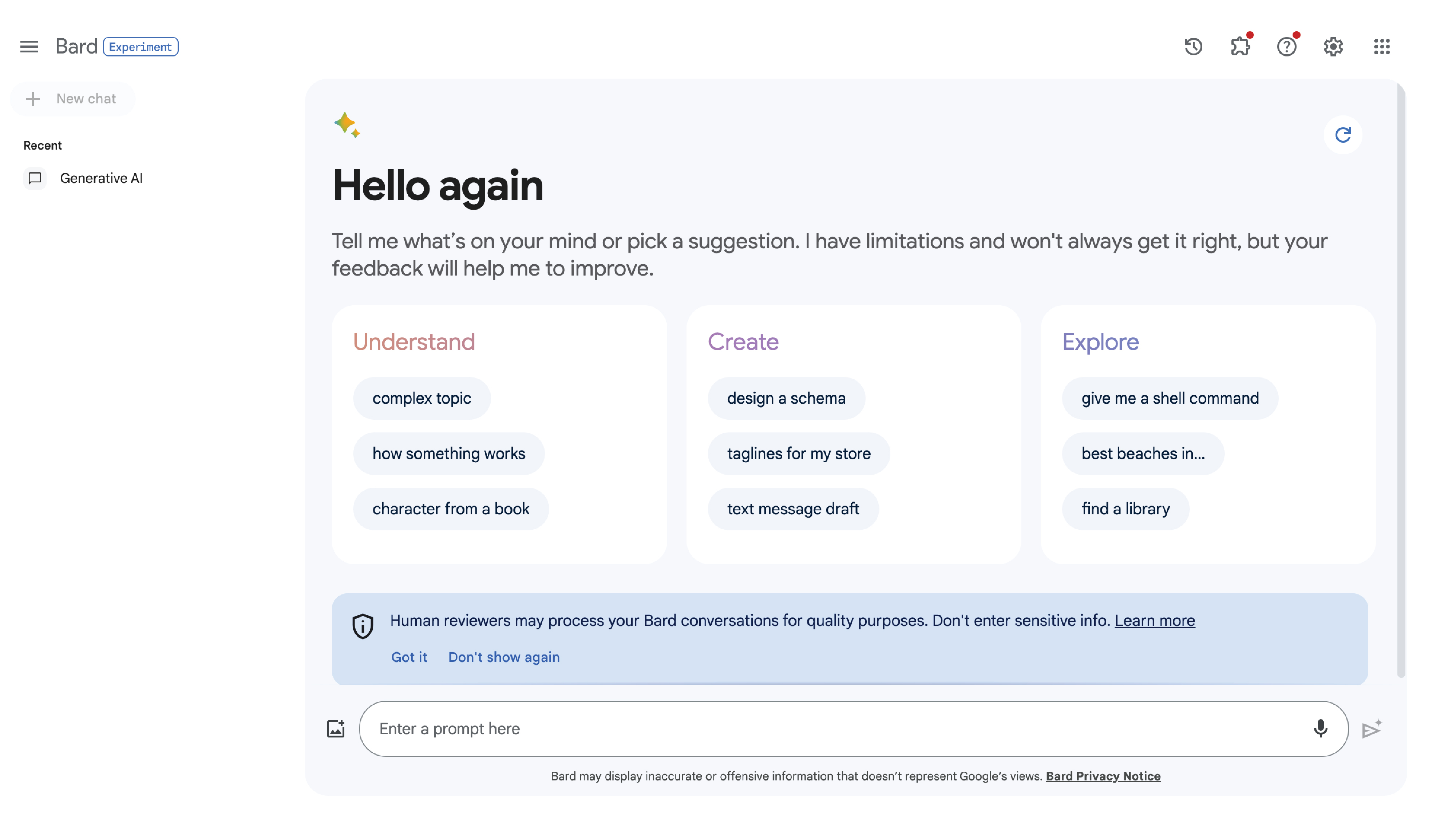
Google pioneered transformer AI techniques for processing various types of content and open-sourced some models for researchers but didn't provide a public interface. Microsoft's integration of GPT into Bing prompted Google to hastily release Google Bard, a public chatbot using a lightweight LaMDA model.
The rushed debut of Bard led to a significant stock price drop when it inaccurately claimed the Webb telescope discovered a planet in a foreign solar system. Microsoft and ChatGPT also faced early implementation challenges with inaccurate results and erratic behavior. Google has now introduced an improved Bard version, powered by PaLM 2, enhancing efficiency and visual responses to user queries.
What are the use cases for generative AI?
Thanks to ground-breaking innovations like GPT, generative AI has a wide range of applications and is usable by people from many walks of life. Some use cases of generative AI include:
- Using chatbots to improve customer service and technical support.
- Implementing deepfake technology to replicate individuals, including specific personalities.
- Improving dubbing for multilingual movies and educational materials.
- Composing email replies, dating profiles, CVs, and academic papers.
- Generating lifelike art in distinct artistic styles.
- Developing compelling product demonstration videos.
- Suggest novel drug compounds for experimental testing.
- Architecting physical products and structures.
- Streamlining innovative chip designs.
- Composing music with a specific mood or style.
What are the benefits of generative AI?
Numerous business domains can benefit greatly from the application of generative AI. Existing content can be easier to perceive and comprehend, and new content can be generated automatically. Developers are investigating how generative AI may enhance current workflows or completely transform operations to benefit from the technology.
The following are some potential advantages of applying generative AI:
- Streamlining content generation through automation.
- Minimizing email response efforts.
- Enhancing responses to technical inquiries.
- Generating lifelike depictions of individuals.
- Condensing intricate data into a cohesive storyline.
- Simplifying content creation in a specific style.
What are the limitations of generative AI?
The numerous drawbacks of generative AI are starkly illustrated by early implementations. The precise methods utilized to achieve different use cases are the cause of some of the difficulties that generative AI poses. A synopsis of a complicated subject, for instance, reads more easily than an explanation with a variety of sources to back up the main ideas. However, the summary's readability comes at the expense of the user's ability to verify the source of the information.
When developing or using a generative AI app, keep in mind the following restrictions:
- Source identification is not always guaranteed.
- Assessing bias in original sources can pose challenges.
- Realistic-sounding content complicates the detection of inaccuracies.
- Navigating new circumstances can be perplexing.
- Results may downplay bias, prejudice, and hatred.
Why does generative AI matter?
If you haven't already realized it, artificial intelligence (AI) is changing how we operate in a wide range of fields, from entertainment to healthcare to life science, and entertainment. Complex problems can be resolved by it fairly immediately. Suddenly, tasks that require imagination and originality can now be generated immediately by machines. In addition to job stability, this has undoubtedly created issues about bias in training data, misuse in the production of false content, ownership, and data privacy.
Closing Thoughts - The Future of Generative AI
With numerous ground-breaking uses in a wide range of industries, generative AI has shown to be a potent technology. Generative AI has the capacity to produce complex and individualized outputs that can facilitate smarter and more effective labor across a variety of industries, from content creation to healthcare. The way we utilize its potential will ultimately determine how generative AI is used in the workplace.
More creative and significant use cases for the technology are anticipated to emerge as it continues to develop and mature. Organizations and individuals will need to keep up with the most recent discoveries in AI as it develops in order to make sure that they are employing generative AI in a responsible and ethical way. For the latest on Gen AI and the future of development in Life Science, keep an eye on Agilisium's blog!